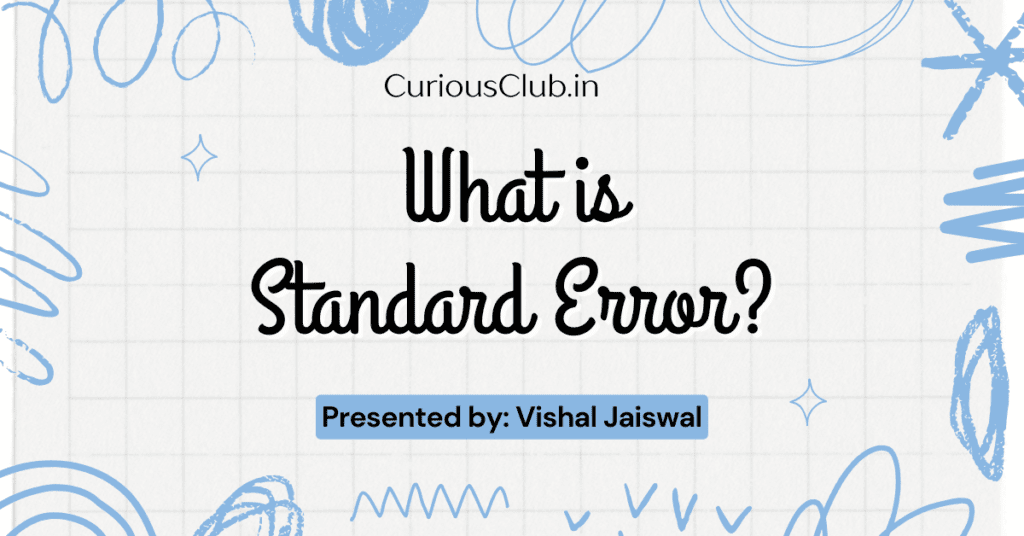
In this article we will understand everything about what is Standard Error, we will be starting with understanding the basics needed for Standard Error and then move on to what is Standard Error, how and why is it used and how to calculate Standard Error.
What is Population?
Population: Population represents complete set of individual or data points. Imagine the entire group you are interested to study, this could be all students in India, All employees of an organization or all visitor of a website in one month. gathering data from entire population can be challenging or even impossible.
What is Sample?
Sample: Since working with entire population is challenging, we rely on samples. A sample is a smaller subset of individuals or data points that are drawn from population, sample data is chosen randomly to make sure that it represents population accurately. For example, if you want to understand the average income of students in India, you might survey a random sample of students from different cities and educational backgrounds.
What is Sampling Distribution?
Since studying an entire population can be challenging, we rely on samples to understand its characteristics. We derive statistics (like mean, median) from these samples. The distribution of these sample statistics is called a sampling distribution.
For example, imagine you want to know the average weight of all newborn babies in a hospital. It is not possible that you will be able to weigh each baby born. So, you take a sample, let’s say you take weights if 50 newborns. You take the average weight of these babies (we will call it sample mean).
Imagine you repeat this process 20 times, each time you take a sample weight of 50 babies calculating their average weight. Each time you do this you get a slightly different sample mean, because the babies you pick will be different. All these different sample means would form a sampling distribution.
The sampling distribution shows the spread of possible average weights you could get by taking many random samples of the same size (50 babies in this example) from the same population (all newborns in the hospital).
What is the relationship between Sampling Distribution and Population Distribution?
The sampling distribution and population distribution are closely related but represent different aspects of data analysis. Imagine the population distribution as the entire picture you’re trying to understand, and the sampling distribution as a single snapshot of a small part of that picture.
Because they represent the same data population distribution will influence sampling distribution, for example, if the population distribution is skewed sampling distribution will also be skewed especially for smaller sample sizes. However, the central limit theorem tells us, as the sample size increases the distribution of the averages of those samples will tends towards normal distribution, regardless of the shape of population distribution.
What is Standard Error?
Standard Error is a statistical term used to represent the variability of estimates (mean, median, mode etc.) derived from samples to estimates derived from population. It tells how much the estimates can vary from population.
What is Standard Error of Mean?
Standard error of mean (SEM) represents the variability between population mean and sample mean. It simply indicates if you were to take a new set of samples, how much the mean of new sample may vary from previous sample given both samples are from same population.
What is the importance of Standard Error?
Standard error estimates how well a sample represents the population, in practical scenarios we will almost never have the population data. In such a case it is important that the sample represents the population accurately. This is where importance of standard error comes to play. Standard error allows you to measure the variance.
A high value for standard error shows that sample means are widely spread around the population mean. A low value for standard error shows that sample means are closely distributed around the population mean.
What is Central Limit Theorem?
The Central Limit Theorem describes the behavior of sampling distributions of means. The CLT states that as the sample size increases, the sampling distribution of the means of random samples tends towards a normal distribution (bell-shaped curve), regardless of the distribution of original population.
The statisticians have determined that, the theory of CLT proves correct when sample size reaches to greater than or equal to 30. Sample size >= 30.
How to calculate Standard Error?
The calculation of standard error depends on the specific statistic you are interested to estimate. Here is a breakdown of how to calculate standard error:
General Steps for Calculating Standard Error:
- Define the Statistic: Identify the statistic you want to estimate (e.g., mean, median, proportion, etc.).
- Sample Size: Determine the sample size (n) used to calculate the statistic.
- Population Standard Deviation (Optional): If you know the population standard deviation (σ), use it for more accurate calculations.
- Sample Standard Deviation (Alternative): If the population standard deviation is unknown, estimate it using the sample standard deviation (s) calculated from your sample data (explained in methods for specific estimates).
- Apply the Specific Formula: Use the appropriate formula based on the chosen statistic (mean, median, proportion) and available information.
Calculating Standard Error for Specific Estimates:
Standard Error of the Mean (SEm):
This is the most common type of standard error. It reflects the variability of sample means around the population mean.
Formula (using population standard deviation): This formula for standard error of the mean is generally preferred for accuracy if you have population standard deviation.
SEm = σ / √n
- SEm = Standard error of the mean
- σ = Population standard deviation (known)
- √n = Square root of the sample size (n)
Formula (using sample standard deviation): This formula is a common estimation technique when the population standard deviation is unknown, which is often the case in real-world applications.
SEm = s / √n
- SEm = Standard error of the mean
- s = Sample standard deviation (estimated from sample data)
- √n = Square root of the sample size (n)
Standard Error of the Median (SEMed):
This reflects the variability of medians you would get if you drew many random samples. Calculating SEMed often involves more complex methods like bootstrapping, but the concept remains similar.
- Specific calculation methods for SEMed involve resampling techniques and are beyond the scope of a basic explanation. However, statistical software packages or online tools can handle these calculations.
Standard Error of the Proportion (SEp):
This captures the variability of proportions you would estimate from many random samples.
Formula:
SEp = √(p * (1 - p) / n)
- SEp = Standard error of the proportion
- p = Sample proportion (estimated from your data)
- n = Sample size
We hope you found the information helpful! If you learned something valuable, consider sharing it with your friends, family, and social networks.
Also Read:
- Understanding Poisson Distribution
- Understanding Standard Deviation: A Measure of Spread
- Understanding Normal Distribution and Random Variable
- Understanding Probability
- Understanding Population Variance
Hi, I am Vishal Jaiswal, I have about a decade of experience of working in MNCs like Genpact, Savista, Ingenious. Currently i am working in EXL as a senior quality analyst. Using my writing skills i want to share the experience i have gained and help as many as i can.